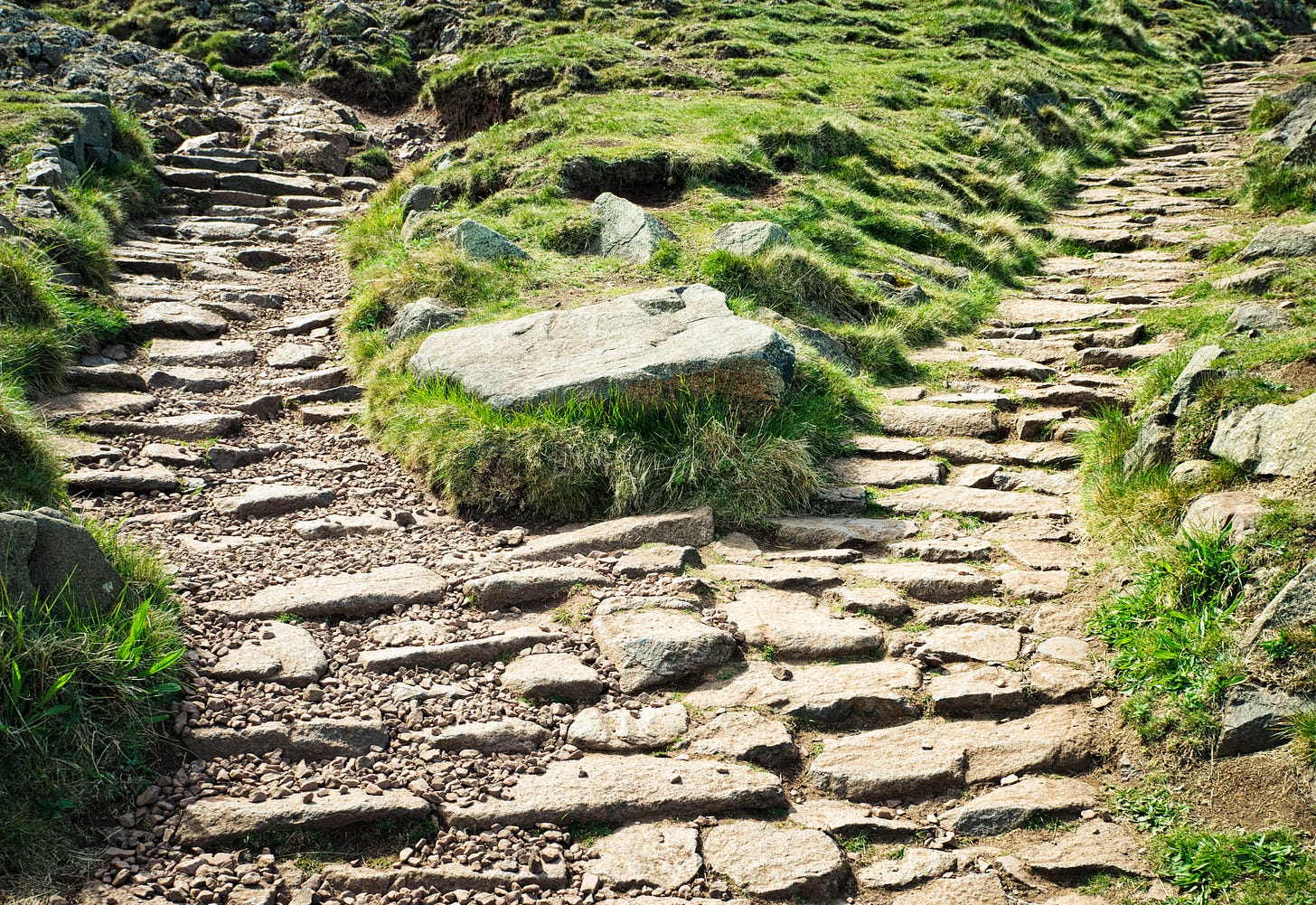
When probability models for electoral forecasts first came onto the scene, I was enthusiastic. I even wrote a piece defending them against attacks by many pundits! I thought such models were an important corrective to the “anything can happen” horse-race reporting that dominated the punditry and the coverage. I thought getting some statistical probabilities out there would allow us to discuss the important policy questions instead.
So why did I just write a piece for the New York Times saying that, for all practical purposes, we should ignore electoral forecasts? Three reasons.
One, what I hoped for is not what happened.The model predictions have just become part of the horse-race coverage. We just spend a lot of time arguing if the models are right.
Two, forecasts themselves are active participants in electoral politics and influence outcomes, something that I had not taken properly into account, but in hindsight is glaringly obvious.
Three, despite great improvements in their presentation I think the current FiveThirtyEight presentation is excellent, compared to the 2016 version, which I criticized for being misleading to the average viewer. And it’s far better than the 2016 presentation by the New York Times model (the needle!), which definitely left a lot to be desired. That said, I still don’t think the general public understands what election forecasts are, what they are not, and what they cannot ever be. I’m not sure there’s a way to overcome this problem. Big numbers still dominate our minds and it is cognitively difficult—really difficult—to truly internalize how much uncertainty is in a model, even if the model suggests “90%” or “96%” odds of Biden winning the election (the current odds for the two big models).
Here’s an excerpt from my New York Times op-ed:
There’s an even more fundamental point to consider about election forecasts and how they differ from weather forecasting. If I read that there is a 20 percent chance of rain and do not take an umbrella, the odds of rain coming down don’t change. Electoral modeling, by contrast, actively affects the way people behave.
In 2016, for example, a letter from the F.B.I. director James Comey telling Congress he had reopened an investigation into Mrs. Clinton’s emails shook up the dynamics of the race with just days left in the campaign. Mr. Comey later acknowledged that his assumption that Mrs. Clinton was going to win was a factor in his decision to send the letter.
Similarly, did Facebook, battered by conservatives before the 2016 election, take a hands-off approach to the proliferation of misinformation on its platform, thinking that Mrs. Clinton’s odds were so favorable that such misinformation made little difference? Did the Obama administration hold off on making public all it knew about Russian meddling, thinking it was better to wait until after Mrs. Clinton’s assumed win, as has been reported?
Indeed, in one study, researchers found that being exposed to a forecasting prediction “increases certainty about an election’s outcome, confuses many, and decreases turnout.” Did many people think like Edward Snowden, who famously tweeted to millions of followers 18 days before Election Day, “There may never be a safer election in which to vote for a third option,” appending a New York Times forecast claiming that Hillary Clinton had a 93 percent chance of victory to his tweet? Did more Clinton voters stay home, thinking their vote wasn’t necessary? Did more people on the fence feel like casting what they thought would be a protest vote for Donald Trump? We’ll never know.
To show you how much I changed my mind, here’s a piece I wrote almost a decade ago, defending models, and specifically those of Nate Silver which were being trashed bly pundits. The piece makes the argument that electoral models are not just necessary, they are good for democracy:
Nate Silver analyzes poll data on the influential FiveThiryEight blog at The New York Times. He crunches polls and other data in an electoral statistical model, and he claims that his work is guided by math, not left or right politics. Yet he's become a whipping boy as election day approaches. His crime? Publishing the results of statistical models that predict President Obama has a 73.6 percent chance of defeating the Republican challenger, Mitt Romney.
“The pollsters tell us what’s happening now," conservative columnist David Brooks told Politico, trashing Silver. "When they start projecting, they’re getting into silly land.” In the same article, MSNBC's Joe Scarborough added, "And anybody that thinks that this race is anything but a tossup right now is such an ideologue, they should be kept away from typewriters, computers, laptops, and microphones for the next 10 days – because they’re jokes.”
David Brooks is mistaken and Joe Scarborough is wrong. Because while pollsters can’t project, statistical models can, and do ... and they do some predictions very well.
My main consideration then was the drive for narrative among journalists, the desire to make things seem closer than they were to keep up the interest among readers and audiences. I still believe the excessive horse-race coverage is wrong: we should be focusing on the policy, the corruption, the implications of elections, rather than spending this much time analyzing polls and making predictions. If interpreted correctly and within their proper context, a probability model is the best way to model odds of many events, yes including presidential elections.
But the phrase “interpreted correctly” is doing a lot of work there, and I was naive and wrong about what would happen once modeling became mainstream. I underestimated the deep human desire to narrativize the outcome and did not foresee how models would become part of the assurance and anxiety-reduction people understandably seek, given the high stakes.
So here we are. As my piece explains, there remains much uncertainty in electoral forecasts, necessarily . Presidential elections are rare events. They are not really repeat events: every four years, we face dramatically different conditions. That makes modeling them even shakier than many other kinds of modeling we do. We don’t have a good, fine-grained grasp of election fundamentals, the way we do for other things we model, like weather. We have sparse data: mostly we have just polls. Our models are especially shaky given that the US is a low voter-turnout country.
If the models and the polls they rely on contain errors and biases, those errors are likely correlated, which can lead to a cascade of error. Remember that most states in the electoral college are winners-take-all. If there is something wrong with the underlying assumptions about voter behavior, that will be true for many states at once. If the shift is enough to change the outcome in a few states, a dramatically different result may be possible, even if the shift itself isn’t that large—just one vote over the line is enough. If there is massive turnout, for example, by Republican voters energized by the assumption their candidate is otherwise losing as predicted by these models, they can easily overwhelm the differences the current polls are measuring. Or if people do not show up on election day because they are demoralized or because they are scared of the pandemic conditions. And on and on and on.
Plus, there’s all the uncertainty around which votes will actually be counted by authorities, and what will happen if the election appears to be close. That’s something the models cannot even touch.
So, am I saying the models are wrong? Not at all. I’m saying that we have no way of knowing they are right or wrong, even after the election happens. They weren’t wrong in 2016. They aren’t wrong now, but they also aren’t right. By their nature, they are not meant to be right or wrong.
Given what we know right now, it’s plausible for either candidate to emerge as the victor. Given that our interest in this election is not a kin to disinterested gambler in betting markets (which is how these odds from the models would be useful), and given the impact the forecasts have on the outcome themselves, the best thing to do is to stop refreshing our web browsers constantly and stop trying to predict, not as a way to return to pointless horse-race coverage the punditry practiced before the models, but as a call to focus on the substance. This is an election, not a sports match, and we are not spectators or gamblers.
Despite all the math in these models, they cannot reduce the anxiety many people feel, and perhaps that’s a good thing. One of the biggest problems in this country, the root of much of our failings, is that our elections are low-turnout—for many reasons, some of which are intentional and some of which stem from the way our politics has long been broken. Feeling the depth of the stakes, and all the anxiety it entails, may well be the first step towards digging out of this malaise. We should want people to vote as if anything is plausible—because it is.
As a computational modeler who specializes in acknowledging and quantifying "uncertainty" (I even recently published a Bayesian COVID model for US states), I have actually recently been wondering about the ethical implications of the entire modeling/prediction/uncertainty quantification endeavor. Part of my dilemma stems from the long-standing tactic of special interests ranging from tobacco to the chemical industry to the fossil fuel industry in "playing up" the uncertainty -- i.e., manufacturing "doubt" -- and wondering whether I've becoming unwittingly complicit. Now, as you mention, having election predictions that predict a "sure thing" can actually have the opposite effect and thereby destroy its own prediction... it's like perverse reversal of a Greek tragedy where trying to avoid a prediction ends up causing it -- here making the prediction can actually undo it... It seems you are suggesting that before endeavoring on a modeling or prediction exercise, we need to consider not only how to effectively communicate the results (which has improved over the years), but what kind of "feedback" making the predictions will have on the predictions themselves? Kind of like the quantum mechanics idea that you can't measure something without disturbing the system? But couldn't you try to model that too (just like they do in quantum mechanics)?
Don't feel too bad about getting it wrong a decade ago, you were a young, smart technophile, and the models were a shiny, smart object. I've always had a skeptical disdain for those models (yeah, the stupid NYT needle was the worst), but then again, I'm not a technophile.
The most informative polls for me are those that have a robust N, and give a +/- error rate.
I like your concept of not being a spectator or a gambler, but an acting participant. This year, I'm a little of all three. The news as of Friday morning that Harris County TX early voting had already exceeded all voting in 2016 seemed more significant that any close poll could be. If a low turnout state was now going to turn out big, the data in that poll seems of less importance than the poll's notion of who a likely voter would be.
Hearing that, I put down a friendly, and maybe aspirational, $5 bet on Texas going blue.